I. INTRODUCTION
Access to quality healthcare is a fundamental right for every citizen. However, in China, the healthcare environment is marked by an imbalance in the distribution of healthcare resources between urban and rural areas, resulting in a heavy strain on healthcare resources [1]. As a consequence, patients face long registration, waiting, and payment times, along with short treatment times [2]. Difficulty in accessing medical visits has become one of the most pressing issues facing the Chinese healthcare system, according to Chinese public opinion polls [3]. In response, China has been implementing reforms aimed at providing accessible healthcare to all citizens. However, limited resources pose a challenge for hospitals to provide one-to-one service for every patient. As a result, health care escorts have become increasingly common in assisting patients during medical visits.
A medical visit can be a daunting experience for many patients, especially those who are anxious, frail, or have complex medical needs. Health care escorts provide emotional, informational, and practical support. Schilling et al. [4] demonstrated that health care escorts have a positive impact on patients' experiences during medical visits, acting as a "third person" to enhance efficiency and communication between patients and healthcare providers.
In response to the needs of patients who lack companions for medical visits, the private sector in China has developed health care escort services. These services include transportation, registration assistance, escorting patients to their appointments, and collecting medication.
The proliferation of mobile technology has led to the emergence of various types of mobile health (mHealth) applications, including mobile applications of health care escort services (MA-HCES). These MA-HCES provide an innovative solution to the challenges faced by patients in accessing healthcare. MA-HCES offers a range of services, such as home pickups, queuing for registration, face-to-face consultations, medication collection, report retrieval, and waiting for patient procedures. Despite the potential bene fits of MA-HCES and the growing demand for health care escort services, their adoption in the market remains low, with limited registration for the service. The challenges faced by MA-HCES in the market suggest that factors are hindering the behavioral intention to use them, including barriers to accessing technology, concerns about the privacy and security of personal health information, and cultural perceptions regarding healthcare.
The rise of single-person households has significant implications for various aspects of life, including consumption patterns, healthcare, and personal welfare [5]. The increasing number of young people living alone in urban areas is particularly noteworthy, and they encounter difficulties in accessing healthcare services. The advent of MA-HCES presents a promising solution to address this issue, yet its adoption among young people in single-person households remains low. To address this research gap, the study aims to develop a model that examines the factors contributing to the behavioral intention to use MA-HCES among young single-person households.
To address this research gap, this study draws on the Theory of planned behavior (TPB) [6], which has been widely used in mHealth research to examine the factors influencing the acceptance and use of technology. TPB posits that behavioral intentions (BI) predict an individual's behavior, and these intentions are determined by subjective norms (SN), attitudes (AT), and perceived behavioral control (PBC). In addition to this, 2 other factors were focused on in this study. In the mHealth domain, privacy concerns and emotional preferences were shown to be important factors influencing behavioral intentions and attitudes respectively [7-8]. Table 1 demonstrates the definition of the above factors.
Items | Definition | Scoure |
---|---|---|
Subject norm | The perceived social pressure to perform or not to perform the behavior. | [6] |
Perceived behavioral control | The subjective degree of control over the performance of the behavior itself. | [6] |
Attitude | The degree to which a person has a favorable or unfavorable evaluation or appraisal of the behavior in question. | [6] |
Privacy concern | Concern about the extent to which personal privacy is exposed to others. | [7] |
Emotional preference | The emotions that motivate individuals to perform the behavior. | [8] |
Behavioral intention | The extent to which an individual will perform the behavior. | [6] |
Therefore, this study hypothesized that subjective norms, perceived behavioral control, and attitudes would predict the behavioral intentions of young single households when using MA-HCES. Privacy concerns and affective preferences were also included as variables to further investigate the factors influencing the use of MA-HCES.
The study proceeds with a review of relevant literature, presents hypotheses and a research model, and describes the methodology including participant recruitment, data collection, and data analysis. The results and their implications are discussed, and the study concludes with conclusions and future research directions.
II. LITERATURE REVIEW AND RESEARCH HYPOTHESES
Mobile health (mHealth) services have emerged as a promising approach to providing healthcare services through mobile devices [9], and have received increasing attention in recent years [10]. The services offered by mHealth fall into 3 categories: (a) diagnosis, treatment, and disease management services, (b) disease monitoring and prevention services, and (c) procedural services to improve the healthcare process [11]. Within this framework, MA-HCES falls under the third category, aiming to enhance patients' healthcare experiences by escorting them to medical appointments and assisting them with various tasks during their visits [12]. MA-HCES is a unique type of appointment-based service that incorporates Internet technology to provide patients with comprehensive care during medical visits, including home delivery, registration queuing, face-to-face consultations, medication and report pick-up, and waiting for patient procedures. The service process of MA-HCES is shown in Fig. 1.
In recent years, several private organizations in China have launched MA-HCES to meet the growing demand for health care escorts. The Epeizhen app was the first MA-HCES launched in early 2015, followed by other similar apps such as the Enjoy Accompany app (https://www.huachengenjoy.com/), the You Jia app (https://www.yjdjk.com/), the Chengyi app (https://www.orange1.com.cn/), the Xiao Ju app (http://www.xjpeizhen.com/), the An Xin app (https://axpeizhen.com/), among others. Additionally, some Internet hospital apps, such as Ping An Good Doctor (https://www.yjdjk.com/), have also added health care escort services to their offerings. Although the market for MA-HCES was slow to take off initially due to low demand and understanding, the COVID-19 pandemic and an aging population have led to a surge in demand, demonstrating the potential for such services.
Compared to traditional medical visit activities, MA-HCES can improve the efficiency of medical visits, enhance the healthcare experience, and provide valuable social support for patients. For instance, the Enjoy Accompany app, the Cheng Yi app, and the Epeizhen app are among the top three apps in the MA-HCES market. The Enjoy Accompany app has been downloaded more than 103,000 times within two years of its launch. This app will serve as a practical case in this study for the questionnaire.
Theory of planned behavior (TPB), which is a useful theory for explaining technology adoption behavior by considering both individual and social influences. According to the TPB, behavioral intention predicts target behavior and is influenced by subjective norm, attitude, and perceived behavioral control. The theory has been applied in research on consumer behavior, sustainability behavior, healthy exercise behavior, and propensity to use mHealth.
TPB considers both internal and external factors that influence an individual's behavioral intentions and external control conditions that are not influenced by individual in-tentions. The use of mHealth is often influenced by a combination of internal and external factors, making the TPB suitable for application in mHealth research.
Numerous researchers have applied the TPB in mHealth research, demonstrating its effectiveness in predicting users' behavioral intentions in this domain. Wu et al. [13] employed a combined TPB and TAM to examine healthcare workers' use of mHealth, while Deng et al. [14] used the TPB in an mHealth adoption study. Li et al. [8] added several new factors (perceived level of illness, perceived medical liability, emotional preference, perceived information risk, perceived medical risk, etc.) to the TPB to examine patient influences on the use of online consultation services in online hospitals. Zhang et al. [15] combined the TPB and Protective motivation theory (PMT) by incorporating individual health disparity variables to create a validation model for the PMT of mHealth services. Wang et al. [16] integrated the TPB with the TAM and added three patient-centered factors to examine the factors influencing patients' acceptance of mHealth services.
Although the TPB has been successfully applied in many mHealth studies, there is a lack of research on the behavioral intention of MA-HCES using the TPB. Given the innovative nature of MA-HCES, it is crucial to utilize the TPB to understand the behavioral intention of young single-person households to use the apps. This study can gain insights into the factors that influence the behavioral intention to use MA-HCES and provide recommendations to increase its usage among the target users.
As the use of MA-HCES is still in its early stages, it is challenging to reach a sufficiently large sample of actual users for a survey. Therefore, this study will focus on the factors that influence behavioral intention towards MA-HCES, rather than actual use.
The subjective norm is the perceived social pressure to perform or not perform the behavior. This pressure may come from important people in an individual's life, such as family, friends, colleagues, or partners [17]. When these people who are important to the individual perceive a behavior as positive, this pressure increases their motivation to perform it. Previous research has shown that subjective norms are a positive and important factor influencing individuals' behavioral intentions in a variety of situations, including mHealth [18-22]. In this study, subjective norm is defined as the perceived social pressure that an individual experiences to use MA-HCES. Based on this definition, this study hypothesizes the following:
Perceived behavioral control is defined as an individual's perception of the ease or difficulty of performing a behavior. Perceived behavioral control is stronger when the perceived difficulty is lower in terms of personal desires, resources, skills, abilities, opportunities, and policies [23]. Perceived behavioral control has a positive effect on users' behavioral intentions to use mHealth [8,14]. Specifically, patients' perceptions of the price, technology, and ease of operation of mHealth applications can significantly influence their perceived behavioral control and subsequent intention to use them. In this study, perceived behavioral control is defined as the degree to which individuals feel confident in using the MA-HCES. Based on the above literature, the following hypothesis is formulated:
Attitude is an individual's overall evaluation of a particular behavior. Attitudes towards a particular behavior are a fundamental determinant of behavioral intentions. The more positive the attitude towards the behavior, the more likely it is that the behavior will be performed [24]. In the context of mHealth, attitudes have been found to be an important predictor of behavioral intention [8,16,25]. For the purpose of this study, attitudes are defined as individuals' evaluations of MA-HCES as positive or negative. Based on this definition, the following hypotheses are proposed:
Privacy concern is defined as a concern about the extent to which personal privacy is exposed to others. Individuals are more concerned when more personal privacy is exposed by performing a particular behavior [26]. Privacy concerns have been identified as a critical factor influencing attitudes and behavioral intention towards mHealth [27-32]. Younger potential users, in particular, place greater value on privacy issues than older potential users. MA-HCES collects private information including the patient's name, contact details, personal health status, etc. As MA-HCES are newly developed, users may be skeptical about the level of privacy protection provided by service providers, leading to concerns about potential privacy violations. For the purposes of this study, privacy concerns refer to the degree of invasion of user privacy that may be perceived by using MA-HCES. Based on this, the following hypotheses have been formulated:
Emotional play a critical role in shaping human behavior [33]. However, the TPB has been criticized for excluding affective processes from its framework [34]. Although attitudes, a key component of the TPB, are thought to have an affective component [35], it can be difficult to disentangle this component from the cognitive and behavioral components of attitudes [36]. Therefore an attempt was made to add an element of affective preference to the research model based on TPB.
Emotional preferences are defined as the emotions that motivate an individual to perform a behavior. When individuals have more positive emotions, they are more likely to facilitate the behavior. In the context of mHealth, previous research has found that emotional preferences influence behavioral intentions and attitudes [37]. In this study, emotional preferences are defined as the emotions that motivate individuals to use the MA-HCES. Therefore, this study seeks to integrate emotional preferences as a component of the TPB with the following hypotheses:
H7. Emotional preference positively influences the attitude of single-person households to use MA-HCES.
H8. Emotional preference positively influences the behavioral intention of single-person households to use MA-HCES.
In accordance with the foregoing hypotheses, this study provide the research model in Fig. 2.
III. MATERIALS AND METHODS
To test the above hypotheses, a questionnaire was used to collect data from the participants, which was divided into three parts.
The first part included a survey description, a short essay on MA-HCES, and two pictures of the MA-HCES. The purpose of this section was to enhance participants' understanding of MA-HCES. The short essay provided a descripttion of the MA-HCES and discussed its impact on the healthcare industry and patients. The two pictures were screenshots of the Enjoy Accompany app interface (Fig. 3) to help participants visualize MA-HCES.
The second part collected demographic information, such as gender, age, education, occupation, frequency of medical visits, and awareness of MA-HCES.
The third part consisted of 21 items that were adapted from previous studies. The measures for perceived subjective norm, behavioral control, attitude, and behavioral intention were adapted from Deng et al. [14]. The measures for privacy concern were adapted from Guo et al. [27] and Featherman & Pavlou [38], while the measures for emotional preferences were adapted from Li et al. [8]. All items were rated on a seven-point Likert scale ranging from "strongly disagree" (one) to "strongly agree" (seven).
Before conducting the formal questionnaire survey, a review and pretest of the questionnaire were conducted. Five individuals associated with the study were invited to review the questionnaire through personal social media platforms. The group included a healthcare industry manager with one year of experience, two doctors with more than two years of experience, and two MA-HCES escorts with more than one year experience. Based on their feedback, duplicate questions were removed, and the wording of questions that could lead to misunderstandings was revised.
Subsequently, 30 participants were recruited for a pretest in Daxing District, Beijing. The 30 responses received were analyzed for reliability and validity, and both were found to be satisfactory. No further changes to the questionnaire were required. Table 2 presents the final version of the questionnaire.
In February 1th-30th 2023, the survey was conducted and distributed through an online survey system in China, the Wen Juan Xing platform (https://www.wjx.cn). The questionnaire consisted of three parts: (1) an essay on MA-HCES and pictures of the Enjoy Accompany app, (2) demographic information, and (3) scale questions.
This study had specific participant criteria to ensure targeted and applicable research. Firstly, the survey focused on young adults aged 20 to 39 years old who live alone. In China's first- and second-tier cities like Beijing and Shanghai, it is common for single young adults to reside in shared accommodations with strangers. Therefore, these individuals were included in the study as single-person households. Secondly, the study only included participants who had visited a hospital within the last six months. This criterion was implemented to ensure that participants had recent experience with the healthcare system, making them more likely to consider using MA-HCES in the future. Invitations to participate in the study were sent to 420 individuals through WeChat, email, and Weibo. As a token of appreciation for their support, each participant who completed the questionnaire received a payment of RMB 2.
Upon completion of the data collection phase, a total of 365 questionnaires were returned. However, after rigorous screening and quality control measures, only 322 responses were deemed relevant and valuable for the study.
Table 3 shows the demographic details of all 322 surveyees. The gender distribution revealed a majority of male participants, with 55.9% being male and 44.1% being female. The age distribution of participants was diverse, with a notable majority (47.2%) falling between the ages of 25 and 29. This can be attributed to the fact that younger, unmarried individuals tend to live in single-person households. Furthermore, the majority of participants were either employed in the corporate sector (68.3%) or students (22.7%). A significant portion (67.1%) of participants held a bachelor's degree. The majority (59.9%) reported have medical visit on 1-2 times per year. Additionally, only 29.8% of participants were aware of the MA-HCES. Overall, the collected sample in this study exhibited a relatively even distribution across demographic variables and provided a good representation of the participants' characteristics.
The collected questionnaires were subjected to statistical analysis. Firstly, the data was imported into SPSS 25.0, and descriptive statistics were conducted to unveil key demographic information. Subsequently, the study employed structural equation modeling (SEM), a multivariate data analysis method, to test hypotheses. The SEM approach consists of two essential components: a measurement model and a structural model. To ensure the accuracy and validity of the study's results, the measurement model underwent a series of rigorous tests using IBM AMOS v26.0. These tests included reliability and validity analyses, as well as confirmatory factor analysis (CFA) and path analysis, all of which contributed to understanding the underlying relationships between the various factors in the study.
IV. RESULTS
The reliability of a study's measurements is crucial for ensuring the accuracy and validity of its findings. Therefore, Cronbach's alpha, a widely used method for assessing reliability, was employed to examine the reliability of each component in this study. Table 4 presents the total Cron-bach's alpha coefficient is 0.83, and Cronbach's alpha coefficients of all factors ranged between 0.828 and 0.912, all of which are higher than 0.80. This indicates that they are reliable for their respective constructs [39].
Constructs | Cronbach’s alpha | Total Cronbach’s alpha |
---|---|---|
SN | 0.828 | 0.83 |
PBC | 0.889 | |
AT | 0.873 | |
PC | 0.881 | |
EP | 0.893 | |
BI | 0.912 |
Conducting convergent validity tests is an essential step in validating a measurement scale as it allows us to verify whether the empirical data collected aligns with the theoretical framework on which the scale is based. Table 5 presents the standardized loadings (λ), average variance extracted (AVE), and composite reliability (CR) of the constructs.
The analysis results reveal that the (λ) for all constructs, except SN4, ranged from 0.72 to 0.92. Although SN4 (λ=0.67) had a standardized loading slightly below 0.70, it was still retained as a constructed item [40].
The AVE values for the constructs ranged from 0.549 to 0.732 (0.549<AVE<0.732), indicating that the collected data exceeded the recommended acceptance value of 0.50 (AVE>0.5).
Furthermore, the CR values for the constructs ranged from 0.829 to 0.916 (0.5829<CR<0.916), well above the recommended cut-off value of 0.70 (CR>0.7). Taken together, these results provide strong evidence that the measurement scale exhibits excellent convergent validity.
Discriminant validity was examined to ensure that the measures used to assess each construct in the study model were sufficiently distinct from each other. Fornell & Larcker and Laufer et al. [41-42] have proposed that discriminant validity can be established when the square root of the AVE of a construct in the research model is greater than the correlation between that construct and the other constructs. Table 6 presents the results require to test that each construct had a square root AVE greater than 0.741 (✓AVE= 0.741), which was significantly higher than the maximum value of any correlation coefficient, which was 0.438. The result indicates that the measures used to assess each construct were clearly distinguishable from one another, and the structure of the research model exhibits excellent discriminant validity.
SN | PBC | AT | PC | EP | BI | |
---|---|---|---|---|---|---|
SN | 0.741 | |||||
PBC | 0.283 | 0.819 | ||||
AT | 0.221 | 0.299 | 0.86 | |||
PC | 0.007 | −0.007 | −0.114 | 0.839 | ||
EP | 0.054 | 0.263 | 0.188 | −0.007 | 0.848 | |
BI | 0.384 | 0.355 | 0.438 | −0.125 | 0.232 | 0.856 |
Fig. 4 presents the structural model estimation of the theorectical modle. Table 7 presents the results of hypothesis testing.
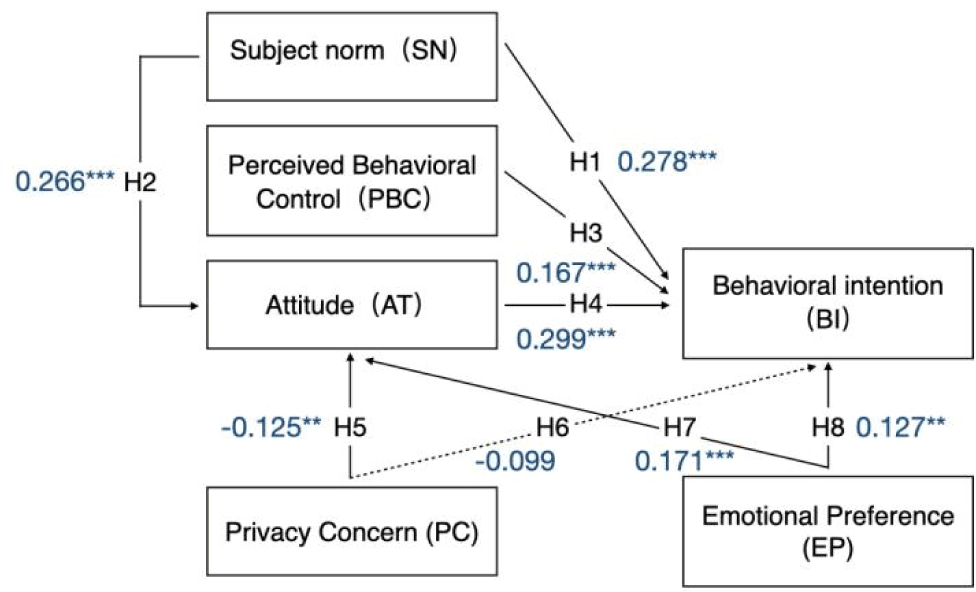
The fit indices were evaluated based on the thresholds specified by Hu and Bentler (1999) [43]. The chi-square fit index x2/df=1.549, (p<0.050), CFI=0.976, RMSEA=0.0041, and SRMR=0.314. These values are within the thresholds, hence the model fit is good.
Firstly, privacy concern had a significant negative effect on attitudes towards the use of MA-HCES among young single-person households, supporting H5 (β=−0.125, **p< 0.01). On the other hand, subjective norm and emotional preference had a significant positive effect on attitude towards the use of MA-HCES among young single-person households, being supported H2 (β=0.266, ***p<0.001) and H7 (β=0.171, ***p<0.001) respectively.
Additionally, subjective norm, perceived behavioral control, and attitude were all significant predictors of behavioral intentions among young single-person households to use MA-HCES, being supported H1 (β=0.278, ***p<0.001), H3 (β=0.167, ***p<0.001), and H4 (β=0.299, ***p<0.001) respectively. Emotional preference also had a significant positive effect on behavioral intention, being supported H8 (β=0.127, **p<0.01).
However, privacy concern was not found to have a significant influence on behavioral intention (β=−0.099, p= 0.059), indicating that H6 was not supported.
V. DISCUSSION
To gain insights into the perceptions of MA-HCES among young single-person households, this study constructed a model to examine the factors influencing their behavioral intention to use the apps. Through analysis of the questionnaire results, several key findings emerged.
Firstly, the findings reveal the important role of attitudes, subjective norms, and perceived behavioral control in influencing behavioral intentions to use MA-HCES among young single-person households, in line with TPB and previous research in the field of mHealth. Notably, attitudes were found to have the strongest positive influence on behavioral intention. This emphasizes the importance of maintaining a positive attitude towards MA-HCES as it significantly increases the likelihood of use by young single-person households. Subjective norms also played an important role in influencing behavioral intentions. Young single-person households often felt pressure from loved ones and friends to use these apps, which was further amplified by the pandemic. In addition, perceived behavioral control was positively correlated with behavioral intention. Young single-person households were more inclined to use MA-HCES when it was easier to operate. Subjective norms had a positive influence on attitudes toward using MA-HCES, which is consistent with previous research by Xu et al. [22]. Young single-person households' attitudes towards these apps were influenced not only by their own perceptions but also by the opinions of those around them.
Secondly, the results showed that although privacy concerns had a negative impact on attitudes towards apps, they did not have a significant influence on behavioral intentions, which is consistent with a previous study by Guo et al. [27] and different from that of Deng et al. [29]. In this study, privacy concerns do not seem to deter users from using the apps. Although the use of MA-HCES does increase the risk of personal privacy invasion and diminishes the positive attitude towards the apps, it is not a major factor that affects user behavior. This could be explained by the Privacy calculus theory (PCT) [44], which states that users tend to weigh the disclosure of their privacy against the potential social benefits and may still choose to use the app if the perceived benefits outweigh privacy concerns. Moreover, it is possible that respondents, who are young single-person households living in first- and second-tier cities, are more educated and have fewer concerns about application providers and MA-HCES escorts.
Lastly, this study hypothesized that a positive emotional preference would lead to a more favorable attitude and stronger intention to use the apps, and the results supported these hypotheses. Emotional factors play a crucial role in shaping young single families' perceptions and behavior towards the app, in line with previous research by Li et al. [8]. the emotional value of MA-HCES is highly valued by young single families. In addition to its practical features in helping with errands and other tasks, the app provides a sense of companionship and emotional support, which is crucial for the well-being and recovery of patients. By involving MA-HCES and its companions in the medical visit process, it is possible to ensure that the emotional needs of the patient are met.
Theoretical contributions of this study are noteworthy, as this is the first empirical study to investigate the behavioral intention to use MA-HCESs, addressing a gap in the existing literature. By incorporating privacy concern and emotional preference into the TPB, this study has constructed a new model that effectively measures the behavioral intention to use MA-HCES. Furthermore, the study expands research on consumption among single-person households by examining MA-HCESs from the perspective of young single-person households.
In practical terms, the study provides valuable insights for MA-HCES service providers. To attract potential and existing users and expand their market, MA-HCESs must meet the needs of their users. Privacy concern emerges as a crucial factor in influencing attitude, and service providers should enhance privacy protection for users by incorporating prompts in the app interface, minimizing the collection of unnecessary personal information, and fostering a positive attitude towards MMAs. Emotional preference also plays a significant role in shaping attitude and behavioral intention, and service providers should prioritize emotional interaction with patients to enhance the user experience. By implementing these recommendations, MA-HCES service providers can improve their services and better cater to the needs of their users.
While this study provides insights into the usage of MA-HCES among young single-person households, there are several limitations to consider, which point toward areas for further investigation.
The survey population for this study was limited to young single-person households, which may not be representative of the broader population of MA-HCES users. During the questionnaire review session with MA-HCES escorts, it was noticed that some young people were using the service to escort their middle-aged or elderly parents for medical visits. Exploring the motivation of adult children to use MA-HCES in escorting their parents could be a potential research avenue for future studies.
Moreover, the majority of participants in this study had limited knowledge of MA-HCES, and the study focused only on their behavioral intention to use them, which may not necessarily reflect their actual adoption. Therefore, future studies should aim to target actual users and investigate their adoption behavior to obtain more accurate results.
Furthermore, this study did not take into account other potential factors that might influence the behavioral intention towards MA-HCES, such as price and an individual's perception of their own health status. Subsequent research could employ other technology acceptance models and incorporate additional variables to investigate the association between these factors and the behavioral intention to use MA-HCES.
VI. CONCLUSION
Mobile applications of health care escort services (MA-HCES) have emerged as a promising solution to the issue of limited healthcare access in China's healthcare system. Despite an increase in registrations for these apps, the market for such services has encountered significant challenges in sustaining its growth. Therefore, it is crucial to identify the key factors driving the behavioral intention of young single-person households to use these apps.
To bridge this research gap, this study developed a novel model that combines the TPB with privacy concerns and emotional preference to identify the factors influencing the behavioral intentions of young single-person households to use the MA-HCES. The results unveiled that subjective norms, perceived behavioral control, attitude, and emotional preference exerted significant effects on the behavioral intentionsof young single-person households to use the MA-HCES. Additionally, the impact of privacy concerns on behavioral intention was found to be negligible.
This finding emphasizes the importance of addressing emotional preference and subjective norms in the design and development of MA-HCES, as these factors significantly shape users' attitudes towards these services. The findings can inform the development of improved apps and offline services, ultimately enhancing the overall patient experience. By considering the identified factors, developers can design user-friendly and effective apps, thereby providing improved solutions for patients during medical visits.