I. INTRODUCTION
Along with the fourth industrial revolution, the deep learning is one of the key technologies that will drastically change our human life in near future. In fact, the concept of deep learning technology has been proposed since the 1950s, and the basic technology was already established in the 1990s of the 1990. The performance of graphics processing units (GPUs) and central processing units (CPUs) that process data will be developed, and big data, which is the material for machine learning, will be in the spotlight after the mid of 2000s.
Big data is pouring into the spotlight. Google ran two deep learning-related teams until 2012, but now runs more than 1,000 artificial intelligence-related projects in search, Gmail, YouTube, Android and Google Maps, while IBM also operates only two projects by 2011. It has recently split into more than 30 teams and is strengthening Watson [1].
As for the creative interactive advertisements that elicit public response, the digital ad industry said, “Many experts are exposed to brands and products for the advertising effects of uniform advertising images and images, but the consumer-participating interactive ads are only more effective.” So how can we get information within content that peoples actually look at carefully and care about? While tracking a person’s eyes is the most accurate method, the current eye-tracking function is quite complex and requires a tracker [2], [3].
In addition to the development of deep learning technology and the new industry that is occurring by combining various service sectors, the company has set the direction of the project with a service that introduces deep running technology to the digital advertising industry, which has been steadily gaining popularity in the advertising industry, and aims to use it to provide interactive window services that increase users’ interest and interest [4], [6], [8], [9].
To detect the eye region, the face detection is very important task such as [11], [12], [13]. From detected face region, we can extract the eye part by using Haar feature. Also, some object tracking methods have been reported in [14], [15], [16]. They employed some deep learning structures to trace the object region more accurately. But if the system can process the detection task in real-time, these tracking approaches are not needed usually.
This paper aims to implement the eye-tracking technology using only the cheap camera without the eye-tracking machine and applies the object recognition technology required for the project using the previously introduced deep running. Also, we aim to provide an interactive window service based on efficient eye detection and pupil tracking technology that recognizes the user eye’s movement and enhances the information of interest.
This paper will be organized as follows: In Section II, we will introduce the developed eye detection and tracking method in detail. Section III will give a proto-type service and some results which has been implemented. In Section V, we will give a concluding remark in final.
II. PROPOSED EYE TRACKING ALGORITHM
Figure 1 shows the overall procedure of the developed scheme. First, we initialize to make the reference (origin) coordinate. Then the view direction is estimated from the extracted eye pupils. Then if the blink is detected, the augmented information is presented. Otherwise, the default information is shown.
The proposed algorithm adopts a face marker detector that extracts and maps 68 point coordinates through deep learning as shown in Fig. 2. Since only the eye area was required for this project, we detect the face area and map it to the input image to identify the area of both eyes using the labeled name instead of the coordinate number of each part.
Once coordinates have been placed on each part of the face through Detect Face Parts, use the labeled name instead of the coordinate number to identify the area of both eyes. Then, we specify each eye as the region-of-interest (ROI). We can use a good and fast segmentation technique to extract the pupil region in [6].
Because this image is blurred, the contrast between light and dark is improved through smoothing and medium-sized filters. After this filtering process, we can find eyes (pupil area) by using the Hough circles function to find a circle. We condition the size of the eye radius and the range of the circle center coordinates so that we find only circles that are likely to be eyeballs. Figure 3 shows the extracted result of the pupil area by the Hough circle transform.
If an experiment is carried out through an eye tracker, the calibration process of setting the reference point as if adjusting the zero of the scale is carried out first. This is a process that helps to produce accurate results, and the algorithm also tracks the user’s eyes after the initialization process is preceded by a baseline [2]. The proposed algorithm also proceeds with initialization, a step in creating a new baseline for a specified number of frames.
During initialization, the user creates a baseline by averaging the center coordinates of the effective circles detected, looking at the center red circle with a pause. Figure 3 shows an example for estimation of view direction vector from the base line point (cross mark).
In this stage, we compute the direction vectors from the reference point. The proposed algorithm determines which area of the image is being viewed through how far the center coordinates of the circle detected after the initialization is away from the baseline. When a human looking at an object, the human eye does not stop but constantly moves its eye pupil [5]. Therefore, the area determined based on the center coordinates of the circle detected in the pupil went up and down the area represented on the screen. It can be shaken.
Therefore, the last five numbers are averaged to determine the number of the area being viewed to stabilize the area. Figure 4 shows an example for estimation of view direction vectors from the base line point (cross mark). In actual, we need to compute the view directions from both the detected eye pupils. We take the average value of the estimated direction vectors.
Eye link detection uses only the left and right eye coordinates of the coordinates mapped to the critical area of the face through Detect face parts. The main principle of detecting eye flicker is to determine that the eyes are closed when the difference in the coordinate values of the vertical axis coordinates (P2 and P6, P3 and P5) of both eyes is not great [10]. To express this in a formula, there is the eye apply ratio (EAR) (top of Figure 5) as:
where pi is the specified position of the detected eye region. This is a fraction of the difference in coordinate values between horizontal and vertical axis coordinates (P1 and P4). In EAR, the difference between the values of the denominators P1 and P4 are very little, so it can be seen that the EAR values are similar when the eye opened, but when the eye is closed, the EAR suddenly becomes smaller as shown in Fig. 5 (bottom). Through experiments, we have set 0.15 as the threshold value to detect the eye blink status.
This eye blink operation is mapped into the click event in the system. That is, the system run an event when the user’s eye is blinked once.
III. PROTO-TYPE SERVICE IMPLEMENTATION
We developed using python and IDLE as an editor. Various libraries were used to receive, process, and display images, but OpenCV was typical to locate each part of the face in real time and refer to the detect face parts using deep learning algorithm for both eyes. The database server was built using the RDS on AWS and MySQL was used as the server engine
Examples of actual use scenarios are as follows: If the program is running and people are detected on the webcam, a greeting message is posted. Then, to indicate that the initialization phase is carried out first at the beginning, a notice is posted as shown in Fig. 6 to indicate that the initialization phase will go through the initialization phase soon. When the guide instruction disappears, a red circle appears in the middle of the screen, as shown in Fig. 7, and the user looks at the circle in front of the screen for a certain period of time.
The location of the user’s eye was divided into large areas and processed to show the area in different colors. Figure 8 shows the building where the eyes are.
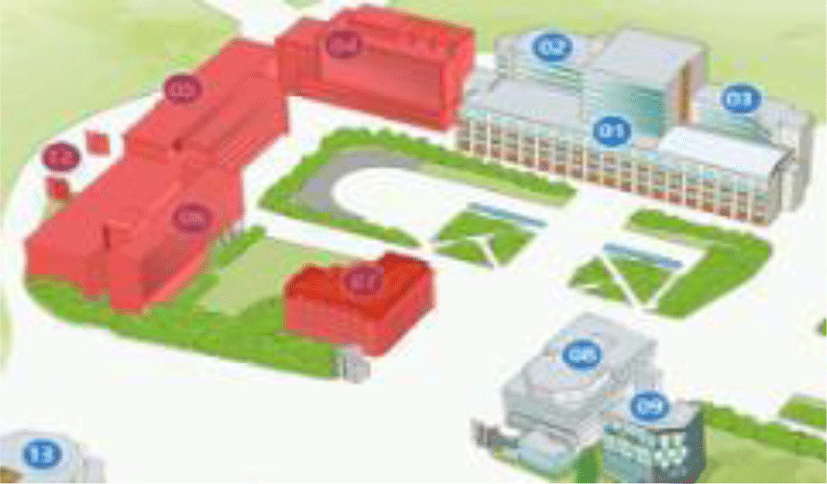
Once the initialization is completed properly, it can be verified that the building is marked red according to the user’s gaze. In addition, when user blinks their eyes while looking at the building for additional information, the designed augmented information about the building will appear on the right for a specified period of time as a statement (Figure 9).
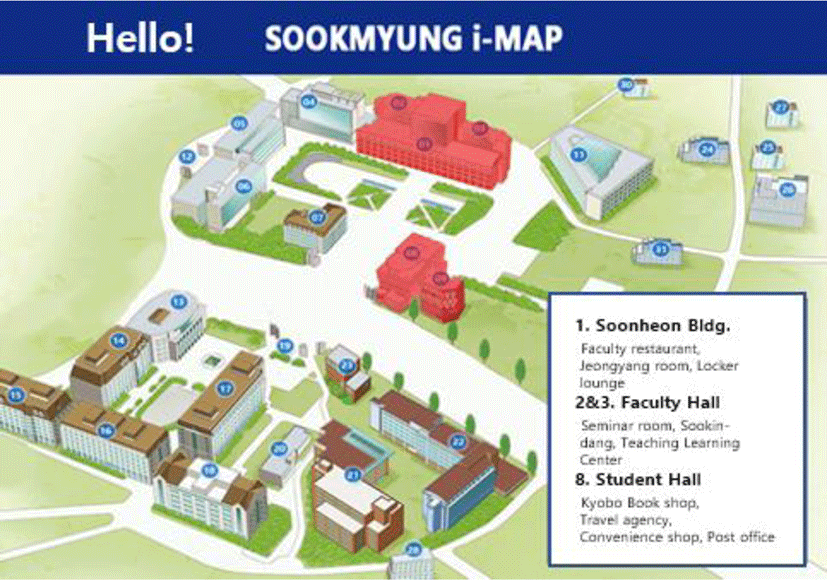
For measuring the recognition accuracy, 24 subjects were recruited to measure the user’s perspective on the service and the accuracy of the service choice. They used the service directly using the developed eyeball tracking algorithm, and each time they determined the selection of a building at a particular location and whether the information was accurately displayed on the screen. Each person tried 3 times for each localization and service event selection. Through this experiment, we measured the accuracy of the exact event.
Table 1 shows the accuracy of the localization and service event selection. The accuracy of the eye pointing was achieved by 88.4% and 85.5% of the service selection accuracy was obtained when a user blinks to select the specific building. The final accuracy of service was 85.5% in the developed system.
Items | Accuracy (%) |
---|---|
Pointing event | 88.4 |
Service selection | 85.5 |
Table 2 shows the consumed time as frame per seconds (FPS). Usual commercial webcam supports the full high-definition (HD) (resolution of 1920x1080). In this resolution, we achieved 22.3 FPS to make final event action. It means that the developed system is able to be applied for real-time system.
Items | FPS | Time per a frame (ms) |
---|---|---|
Processing time | 22.3 | 44.84 |
III. CONCLUSION
In this paper, we have developed an interactive and information augmented service based on the efficient eye tracking algorithm which have the potential to develop into a breakthrough technology that can complement the limitations of the existing expensive eye-tracking technology that relied on sensor or physical tracking hardware. We achieved near real-time eye tracking-based interactive system with 85.5% of the service accuracy.
The developed system can be applied by changing the subject matter to a variety of services and situations, as well as mapping services.